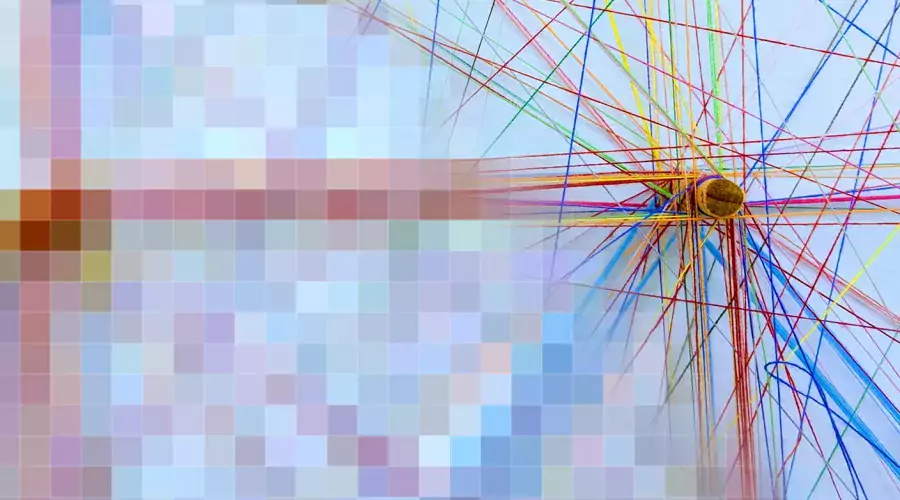
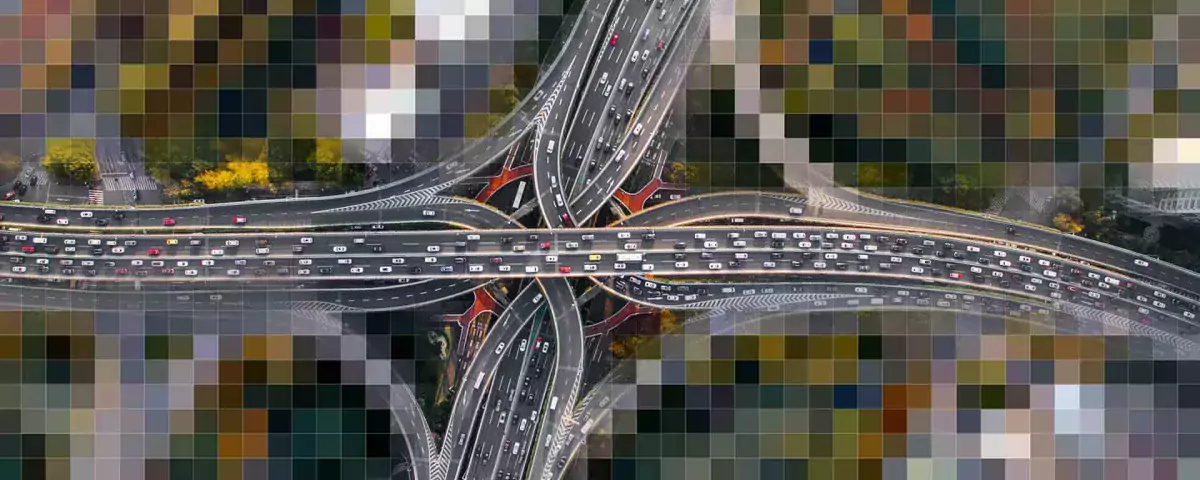
17.07.2024 | Blog Generative AI in the Company: This is How it Works with Data Protection
1.The Challenge of Implementing Generative AI
Companies, public authorities, and municipalities are increasingly exploring how they can use generative AI to relieve their employees and work more efficiently. Pilot projects are being launched, and OpenAI or similar cloud-based providers are being quickly connected and tested. Some even set up their own Large Language Models (LLM) to summarize the organization's documents, research internal information and create new texts from it.
2.Technological Hurdles to Implementation
The IT departments of organizations are usually very ambitious and competent. Tests often work well 80 percent of the time, but difficulties arise with the remaining 20 percent that are required for a complete and production-ready solution. A major challenge here is to consider read and access rights — and to ensure that AI-generated answers do not contain sensitive information that is then released to unauthorized persons.
Data is often duplicated, and no one knows which is the original. In some cases, this data may not be uploaded at all. Others find that features such as auto-completion, follow-up questions, highlighting in documents, curation, and evaluation of answers, etc., which they are used to from other applications, are often missing and would first have to be implemented on a project-specific basis. You would need software or machine learning developers to take the application out of the test phase and into production. This can quickly lead to project costs above 100k for in-house teams or external service providers.
3.Our Solution: Organization-Wide Search with Consideration of Access Rights
At this point, organizations come to us, and we are happy to support them with our standard product that already contains complex features such as those mentioned above. As a specialist in enterprise search, secure searches in an organization's data are our core competence. For over 20 years, we have been enabling companies to find relevant information in their data while automatically considering existing user access rights. This means that every user only finds the information they are authorized to see — starting with the autocomplete suggestions of the classic search. This is an essential prerequisite that ensures that user rights are also taken into account for AI-generated results.
4.The RAG Approach: Combination of Language Model and Intelligent Search
How do we achieve this? We work with the RAG approach (Retrieval-Augmented Generation). This combines a generative AI language model with our intelligent search engine. When queries are made, the AI-based technologies of Enterprise Search retrieve relevant information from the organization's data and make it available to the language model that generates the answer. As a result, the output of the language model is always based on up-to-date information, significantly reducing the risk of hallucinations. Enterprise Search also automatically considers read and access rights to these data. Employees or customers only receive information in their AI-generated responses that they are authorized to see. This automatically fulfills a central data protection requirement that is often overlooked in the current generative AI test euphoria, or deliberately ignored at first because it is very demanding. The iFinder software makes it possible to provide all relevant content, searchable and findable, vectorized — i.e. enriched with the embeddings from the LLM and with the respective access rights.
5.Successful Integration of Existing LLMs
When we tell organizations that we can not only solve their problem with read and access rights, but also use their existing LLM and combine it with our search, they are delighted. Their previous work with the LLM was not in vain, and their requirements for beneficial use of generative AI as efficient employee support are met. Whether the companies bring their own LLM, or we at IntraFind select a suitable LLM for their use cases in consultation with our customers, we support organizations in using their data with generative AI to increase productivity through optimized information provision.
Conclusion
Pilot projects in companies and the public sector show that LLMs and generative AI reduce the workload of employees. Successfully implementing LLM projects is a challenging task for organizations. It makes sense to bring AI specialists on board right from the start, for whom these topics are part of their daily work.
Related articles
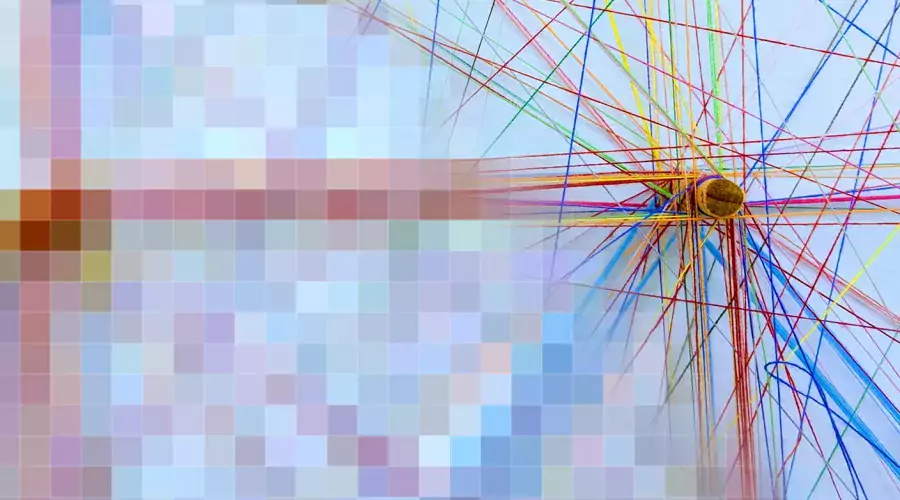
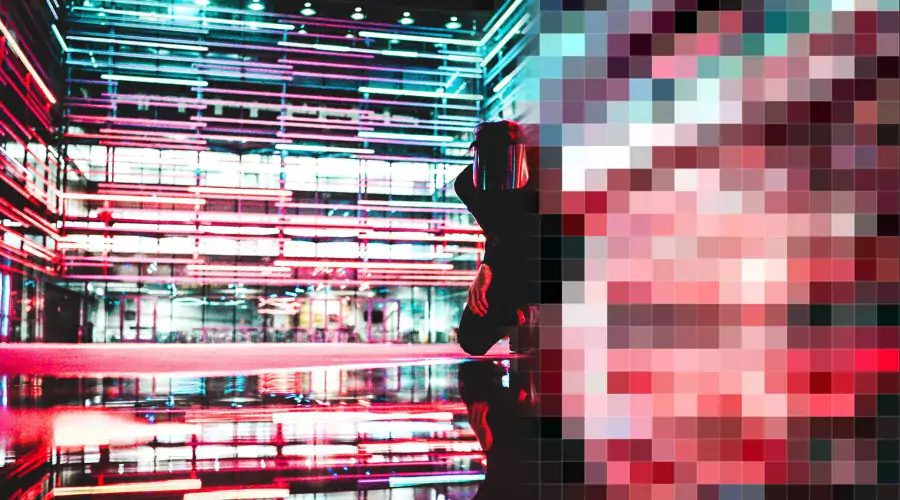
Retrieval Augmented Generation – What's it all about?
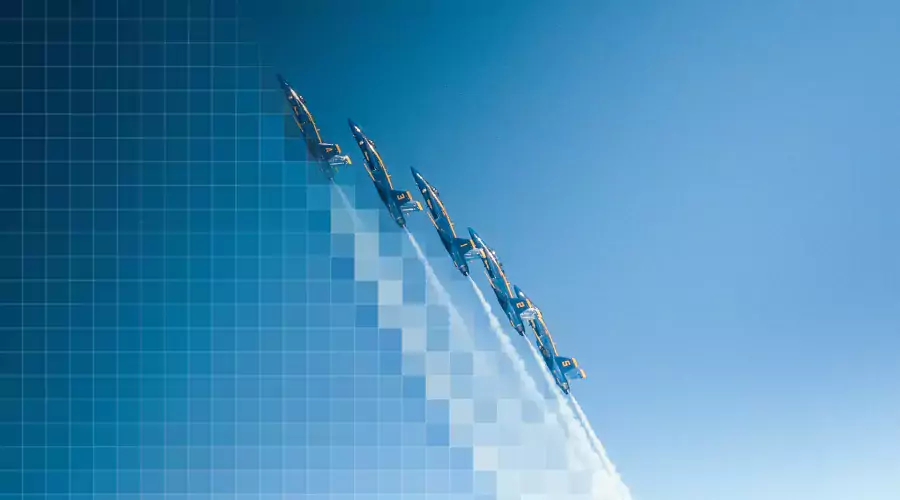
Maximum efficiency: How public authorities and companies benefit from enterprise search with genAI
The author
Daniel Manzke
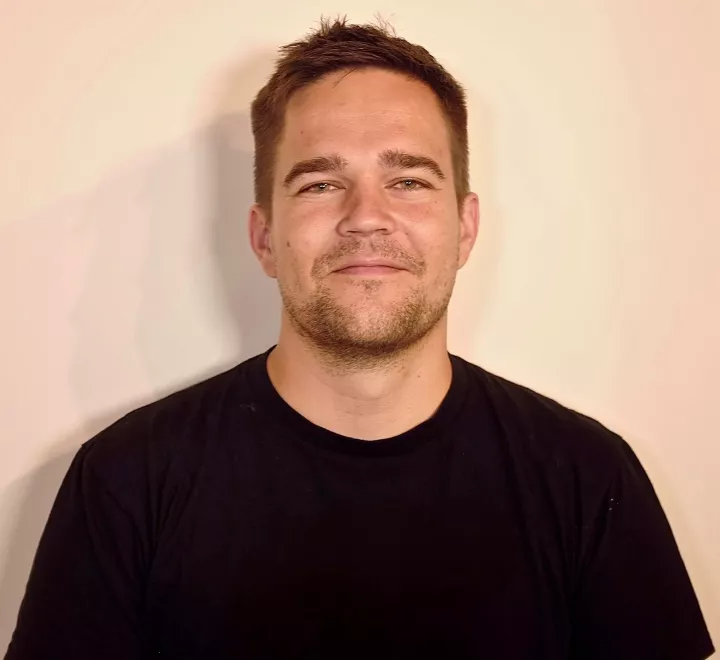