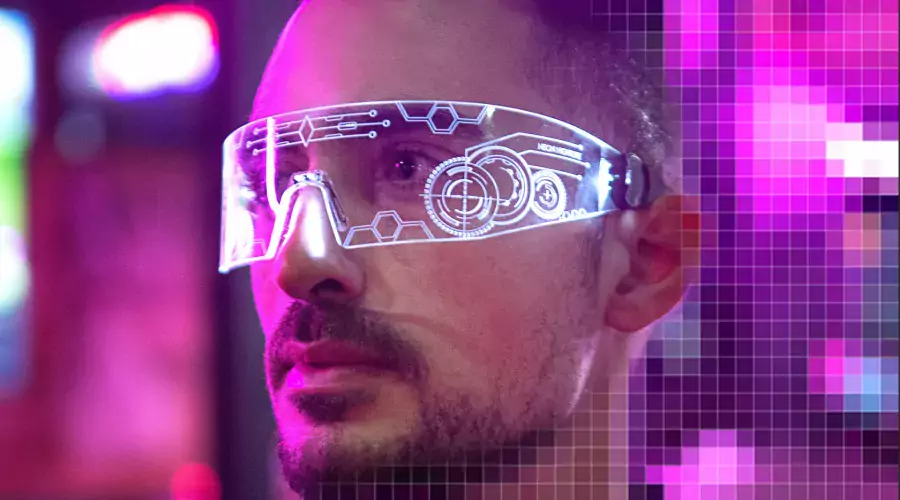
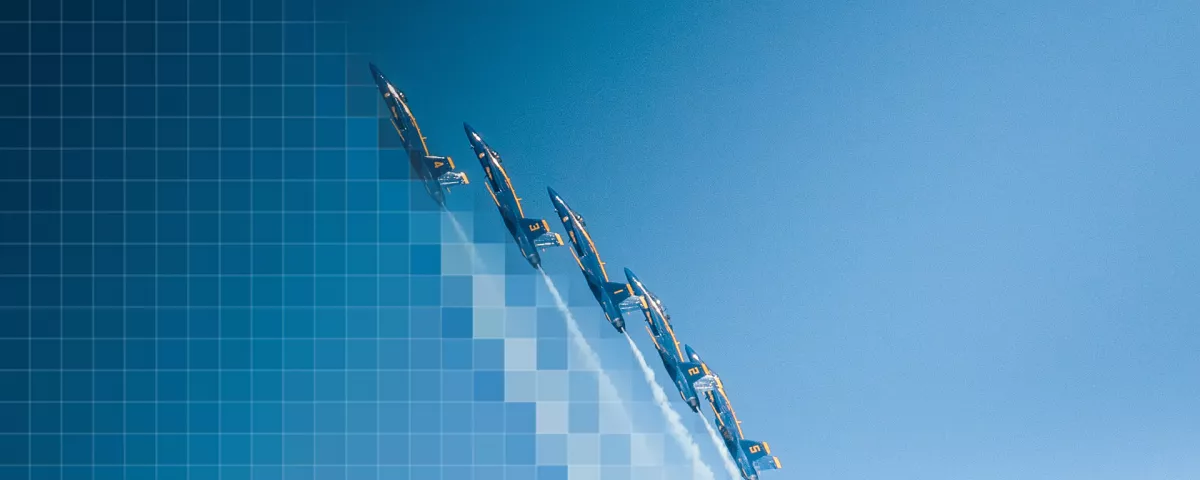
29.09.2023 | Blog Maximum efficiency: How public authorities and companies benefit from enterprise search with generative AI
Large Language Models take organization-wide search to a new level
Since the current AI hype, there has been a lot of talk about Foundation Models, Large Language Models and generative AI. Let's first put these terms in the context of organization-wide information search. What added value do these technologies offer for daily work in companies and government agencies? Does it matter whether the data is stored in the organization's own IT infrastructure or in the cloud? Are there risks involved in using LLMs in companies? For which specific use cases does the use of LLMs make sense?
AI technologies have been used in modern search software for some time. We have been doing this in our iFinder software for 15 years. The new Large Language Models trained on extensive text data open new possibilities for answering questions in natural language and for further developing the search function.
At a glance:
- For classification: Relevant AI terms in the context of enterprise search
- Risks and opportunities of LLMs
- Use language models in organization-wide search without risk
3.1.Summaries and answers
3.2 Data enrichment
3.3.Keyword search und semantic search combined - Enterprise Search with LLMs in practice - examples for more efficiency
1. For classification: Relevant AI terms in the context of enterprise search
To create a common understanding, I would first like to explain some terms from the world of artificial intelligence that are relevant for the search.
Foundation Models
are models that have been trained based on large amounts of data and can be adapted for specialized tasks. This process is often referred to as fine-tuning and the entire process as transfer learning. Foundation Models can handle different data modalities. The first models of this kind emerged in image processing.
Large Language Models (LLMs)
are a subset of Foundation Models that have been specifically trained to process language using text data.
BERT-like models
can learn representations of text (embeddings of data points). These play a major role in search using vector databases, for example. In the context of enterprise search, BERT models play a major role in retrieval, i.e., search itself. Another important use case is enrichment for entity extraction.
Generative models
are able to generate new data. This new data is ideally indistinguishable from data points in the training dataset in terms of statistical characteristics. A prominent example is ChatGPT from OpenAI, a conversational chatbot based on generative models, or LaMDA from Google. In addition, there are good open-source models. These generative AI models are mainly relevant in the context of search for generating answers based on hits; however, we can also use them for data enrichment, i.e., adding (meta)-data to an enterprise dataset to classify it by topics, for example.
Chart: Relevant AI terms in the context of enterprise search
2. Risks and opportunities of LLMs
As with many technological advances, LLMs carry both opportunities and risks. Risks include the generation of false information, privacy issues, and the risk of intellectual property infringement. For example, data points in the training dataset may be "memorized" by the model, and a derived system may therefore violate privacy policies, or illegally reproduce intellectual property, or simply make things up from the model, i.e., hallucinate.
On the other hand, LLMs can dramatically improve the user experience of software systems and machines by enabling more intuitive, human-machine interaction, for example providing answers to questions instead of a list of documents with matching hits. Last, modern AI systems can help automate and streamline text-related tasks, especially in times of demographic challenges. This applies to both the reception and production of texts.
Misinformation and limitations of known models
The tool ChatGPT can answer some questions surprisingly well. For example, it can reproduce the year of the French Revolution. However, this is to some extent a coincidence.
Generative models like ChatGPT generate text word by word from left to right, based on an existing text - the prompt (a "working instruction") - and the text already generated so far. In this process, the models have no actual understanding of the content in the human sense but reproduce learned patterns from massive amounts of text that they have sifted through during training.
This can lead to the models generating false information that looks and sounds like real information. These errors are unavoidable and can be difficult for the user to detect. Therefore, these models are not suitable for answering factual questions.
3. Use language models in organization-wide search without risk
Within the search engine, on the other hand, the use of LLMs offers significant advantages in terms of data security, copyrights and timeliness. Here are some application examples:
3.1. From search engine to answer engine - summarizing documents and generating answers
An ideal use case for generative AI models in the context of enterprise search is the further processing of hits, for example to generate a summary of the found texts parallel to the hit list. We can also use generative models to enter a kind of dialog with search results, i.e., to ask questions and receive answers. The risk of misinformation, as seen with ChatGPT, is massively reduced by this approach. This is because the generative model works with the the organization's own data that are factually correct, and the task is to summarize this information.
We build here on a modern enterprise search engine with its own advantages, i.e., the person searching is presented only with the facts they are permitted to see - an important aspect when using LLMs in an enterprise context.
IntraFind's iFinder search engine brings along generative models (such as AlephAlpha's Luminous) and is capable of running this model on-premises, in organization-owned data centers. This eliminates the problems of data security and copyright that we mentioned above as risks of known models. The advantage is that organizations do not need to build their own expertise and invest the resources to build, maintain or integrate a model themselves. However, it is of course possible to bring your own models or use SaaS LLM services such as Azure OpenAI if desired.
3.2. Data enrichment - optimized solutions for specific use cases
The second option to extend a search engine with LLMs is to enrich documents with so-called extractors (for example extracting entities such as people, companies, etc.) and classify documents by topic.
Enrichment algorithms - whether AI-based or not - that act only at text level have limited access to the document information object. Especially in non-linear documents, extractors operating only at text level reach their limits. Such documents – for example delivery bills, invoices or forms –are not read from top left to bottom right. They contain isolated pieces of content, therefore, the order of the extracted text often does not correspond to semantically related blocks.
This is where so-called multimodal models are used, which consider both text and layout of the document. These can process even non-linear documents precisely and thus offer optimized solutions for specific use cases for layout analysis, optical character recognition (OCR) or intelligent document processing (IDP).
3.3 Keyword search and semantic vector search combined - the best of both worlds for even better results
Semantic search is not a new term, but it is on everyone's lips again. Semantic search can be described as follows: The search engine should find what I mean, not what I type. The goal is to get results that match the intended meaning - even if you don't type the exact term. This is where language models of the representation learning variety come into play, i.e., models that calculate number vectors (so-called embeddings).
This brings us to the third option available to us through the integration of modern language models: enriching the search index with embeddings. Here the documents are enriched analogously to the linguistic enrichment during indexing by means of LLMs.
Searching with embeddings (also referred to as Dense Retrieval or Semantic Vector Search) is an effective tool to overcome the so-called "Vocabulary Mismatch" problem. This means that the search results are more relevant because the search returns hits that match the meaning instead of being based solely on the entered words.
Established search technologies are very advanced and have many advantages. For example, language models can only handle terms to a very limited extent that they have not seen during training and thus do not know. A traditional, lexical search, on the other hand, can do this excellently without having to be trained. Depending on the use case, traditional search methods may thus be more helpful and the use of generative AI may not be necessary. For example: If a user is looking for a presentation on a particular project, they want to find that specific document and not receive an AI-generated answer about it.
iFinder can combine both search methods and use them simultaneously. Thus, it offers a combination of classic keyword search, advanced linguistics, and semantic vector search to achieve the best results.
4. Enterprise Search with LLMs in practice - examples for more efficiency
When organizations deploy a search engine using LLMs, they can increase the efficiency of work processes, improve information access, and increase the quality of generated work results. Here are some examples:
- It is an excellent way to create concise summaries of organizational knowledge, for example from extensive reports, presentations or electronic files, complete with source references. A useful application in public administration is a "note assistant" that supports clerks to create notes. Here, the focus is on generating a summary of the facts, while the assessment and final recommendation remain the human responsibility.
- Chats to get answers from this content are also possible. The LLMs in the search engine work with actual data that can be used to generate an answer, for example: "ask the document / ask the e-file / ask the hit list".
- In general, the topic of question-answering systems is improving enormously in the corporate/government context - these can be internal organizational queries, for example: "How do I call in sick?" as well as chats with customers or citizens.
- In this way, the service of industrial companies can be optimized and made more convenient for users. A concrete use case is the service employee, who can have the AI quickly generate solution suggestions from the extensive technical documentation in the event of a service case, or the chat for customers, which is fed from service documents.
- Completely new options are available for the automatic processing of documents such as invoices, delivery bills or incoming orders (Intelligent Document Processing).
- In general, the topic of knowledge management is raised to a new level - with a more convenient user experience and improved results: Search in combination with generative AI facilitates the onboarding of new employees and it makes the knowledge of former employees accessible, because employees get answers from the curated corporate knowledge.
These are just a few examples from numerous fields of application. In fact, AI opens a wide range of practical opportunities for companies and public authorities. Organizations know their processes best themselves and have an idea of where AI can support them and make them more efficient.
In the context of enterprise search, they benefit from the following:
- They retain full control over their data, whether stored on-premise or in their own cloud.
- In addition, they receive reliable information from trusted organization-owned sources.
Conclusion
With enterprise search that integrates AI language models, businesses and government can unleash the full potential of their data, facilitate effective knowledge discovery, and increase productivity across the organization. In addition to the proven skills of comprehensively finding relevant information from large data sets, there is the ability to succinctly summarize information and generate answers from trusted organizational sources.
As with all projects, before initiating an AI project, you should be clear about what you want to achieve with AI. We invite you to join us in making AI innovations a reality and would be happy to discuss your use case without obligation.
Related articles
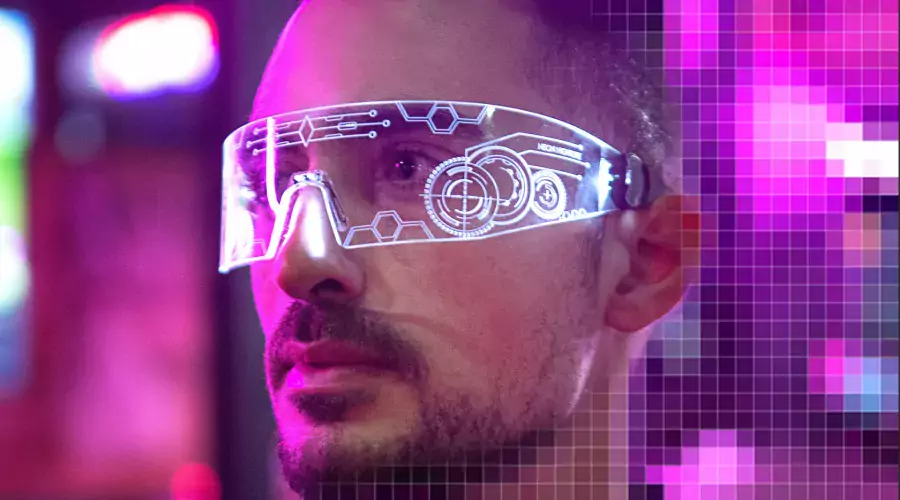
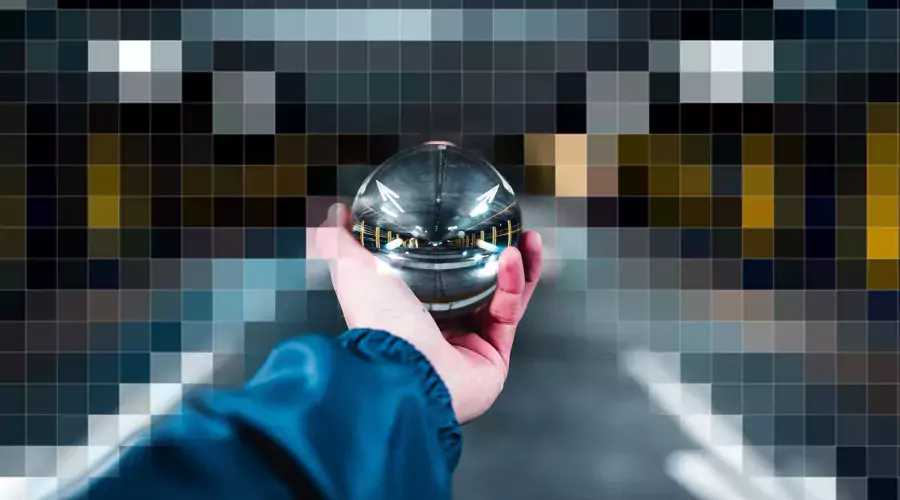
ChatGPT: The Future of Search?
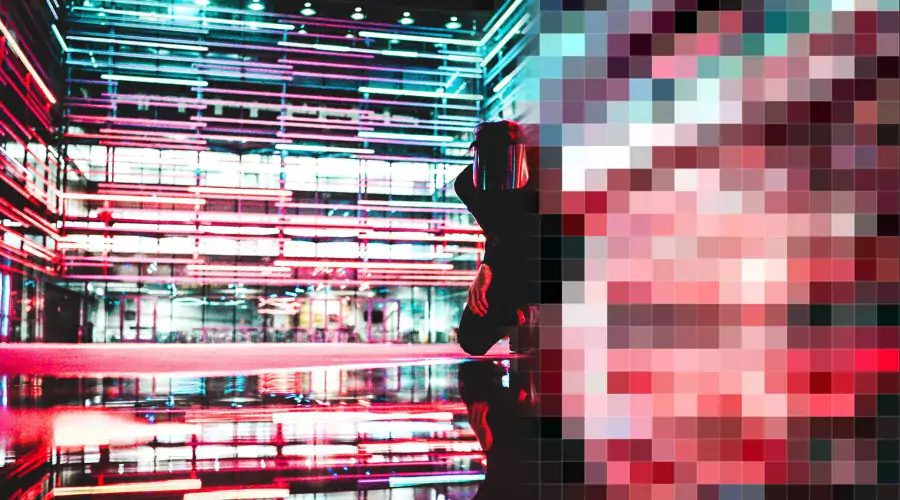
5 points to look out for in your AI projects
The author
Breno Faria
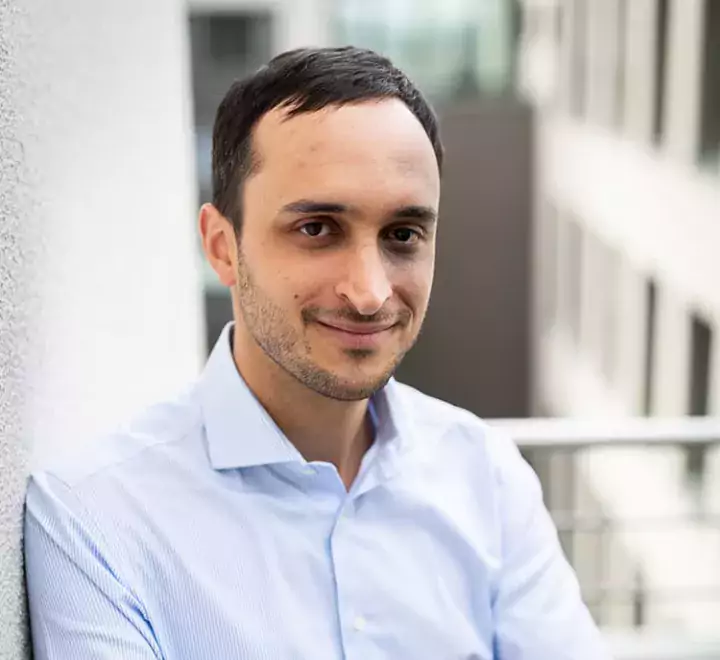