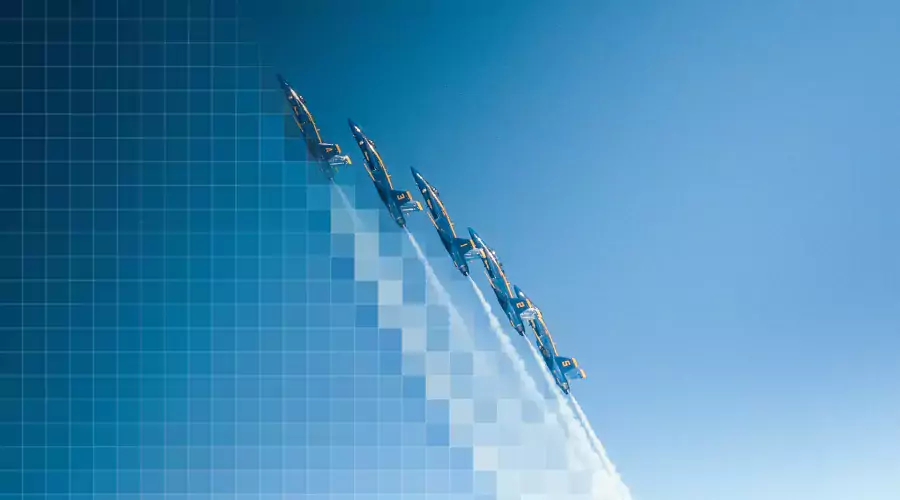
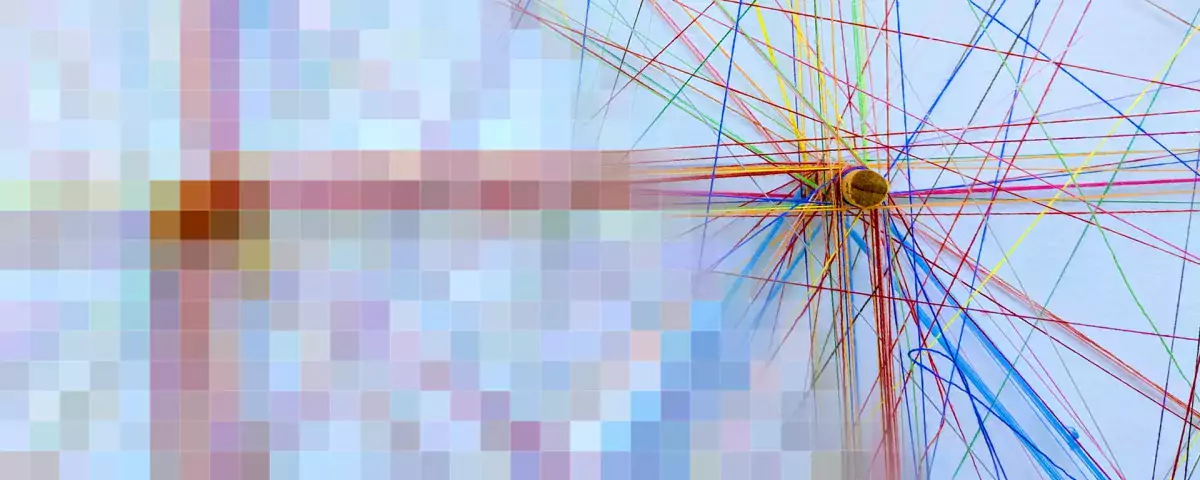
26.03.2024 | Blog Generative AI: From hype to practical application in business
ChatGPT experience in a business environment
Many organizations have already taken the first steps and tested generally accessible generative applications such as ChatGPT. From research to the creation of outlines and presentations to the collection of ideas for marketing texts. The task now is to go beyond the experimental phase of individual departments and, in addition to using generally accessible information, implement specific applications with the organization's own data that accelerate processes and increase efficiency, for example:
- in customer service and helpdesk
- in sales
- for knowledge management
- for administrative processing
- in citizen services
Challenges in integrating corporate knowledge
As studies show, companies do want to use generative AI. One aspect that organizations need to consider in order to benefit from generative AI is the effective and safe use of their own data with Large Language Models (LLMs). LLMs have been trained with a huge amount of general knowledge, but how can they ensure that LLMs also incorporate organization-specific information or industry know-how? This is the only way to optimize customer service or create partially automated memos in public administration. The reliability and accuracy of the output is just as important as ensuring data security and access rights.
The challenges are:
- Include specific knowledge
Companies must ensure that generative AI can access their own knowledge. For example, if they want to support employees in customer service, it must be possible to find article numbers, product information, troubleshooting instructions and solution descriptions. - Receive valid, up-to-date answers and summaries
AI-generated content must meet the specific requirements of an organization and deliver high-quality - and above all correct - output. A targeted retrieval solution is required that incorporates current internal data to identify correct and reliable content and passes this on to a generative LLM. This is crucial, because an LLM only knows the data up to the time of its training. - Ensuring data security and access rights
When using LLMs, organizations must also think about the protection of their data. On the one hand, company knowledge and proprietary data must not be leaked so that AI manufacturers can use it to optimize future generations of LLMs. On the other hand, the authorization structures of the data source systems must ensure that only authorized persons within the organization can access the relevant information. So-called "prompt injections", which can be used to manipulate the functionality of a generative LLM, must also be prevented.
The solution: Retrieval Augmented Generation (RAG) combines LLMs with Enterprise Search
How do you solve the challenge of using company data securely for generative AI? The answer lies in intelligent enterprise search software. This software for organization-wide information search first connects the desired company sources and bundles them in a single search index. The software uses sophisticated AI, machine learning, natural language processing and semantic vector search methods to deliver the best search results. Based on the rights-checked information found in the index, generative AI creates helpful answers or summaries including references. As the language model thus receives valid organization-specific and domain-specific information and data, the risk of hallucination is significantly reduced. This concept is known as Retrieval Augmented Generation (RAG).
| 2. Generative AI makes it possible to use the information found in this way. For example: |
|
|
How does it work technically?
Retrieval Augmented Generation interposes search software between the question and the AI-generated answer
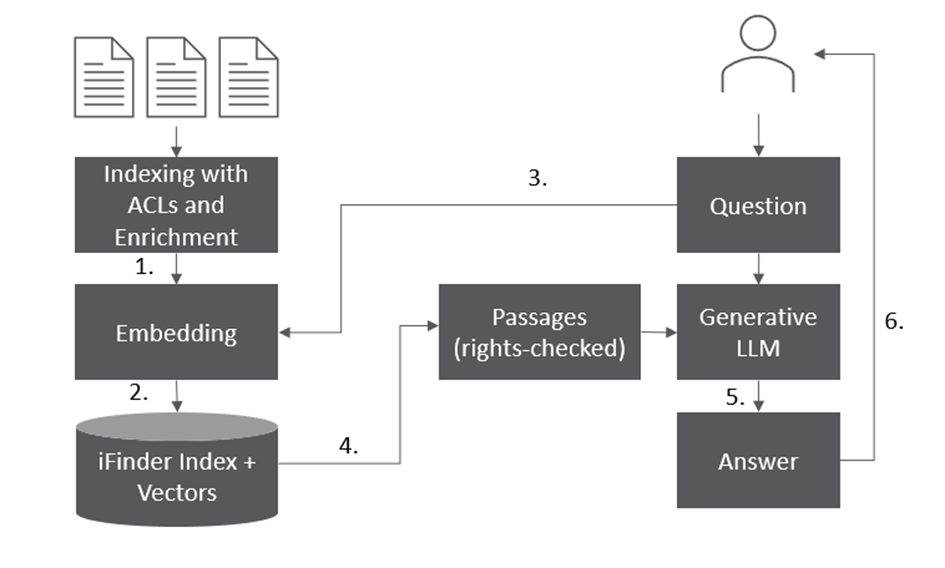
With the help of Retrieval Augmented Generation, large AI language models are combined with enterprise search technology.
Enterprise Search connects all desired data sources via connectors, indexes their data and documents and enriches them during the indexing process. This creates a linguistically normalized full-text index. Additional internal processing splits the documents into text passages and generates numerical representations of the text with so-called embedding LLMs. This process creates vectors (embeddings) that represent the content of a text with hundreds of numerical values. This method enables the software to identify documents or text passages that are closely related to the content of a search query.
If new documents or data are added to the connected data sources, the search software automatically indexes them. This ensures that your index is always up to date. In addition, suitable software provides end-to-end permission management. It takes access rights into account during indexing and automatically applies them when searching and displaying hits to a user. Users only see data in their search results for which they have the appropriate authorization.
Further safety factors when using LLMs
Not only LLM-based chatbots on the internet or on websites, but also LLM-based systems in the company must be protected against misuse by so-called prompt injections: This is done through a series of protective mechanisms that filter manipulative or harmful prompts and prevent the generation of inappropriate or unwanted responses.
It is also important to emphasize that enterprise search products such as IntraFind's iFinder support on-premise LLMs for the creation of embeddings and generative AI, this means they are used on the organization's own servers. Thus, no information in transferred to cloud services - no matter how diligently cloud providers claim the security of their services.
The generative part requires the use of GPU computing power on-premises. However, IntraFind also offers this as an exclusive service (LLM as a Service) for iFinder customers who would initially like to test the possibilities of generative AI without their own GPUs.
How to choose the right Large Language Model?
In addition to the well-known LLMs from large tech companies such as GPT or LaMDA / Gemini, there are other very good proprietary models such as Luminous from the German manufacturer Aleph Alpha. Open-source models also deliver excellent results. Depending on the application, smaller, specialized AI models also often produce the desired results. In addition to the model size, you should also consider the size of the so-called context window of the LLM, this means the amount of text that the model can view or analyze at once.
In the case of proprietary LLMs, the costs incurred should be considered by carefully evaluating the often unpredictable token-based pricing models. After vendor lock-in with one of the platform providers, disillusionment with escalating monthly usage costs in a pricing model that is difficult to calculate is almost inevitable.
Finally, also think about usability. There are models such as ChatGPT that generate the answer fluently or display the complete answer in one fell swoop even after several seconds of waiting.
If companies use generative AI in conjunction with enterprise search software such as IntraFind's iFinder, they don't have to worry about selecting the AI model. We take care of this as a provider, tailored to your use case. If you are already further along and have your own LLM, we can also integrate it into the search.
Conclusion: Valid answers instead of hallucinations à la ChatGPT are possible
Despite the promising benefits of using generative AI, there are challenges in terms of organization-specific information and data security. At IntraFind, we support you in ensuring reliable output and watertight access management. Come to us with your application idea. We will support you in further developing and implementing it.
Related articles
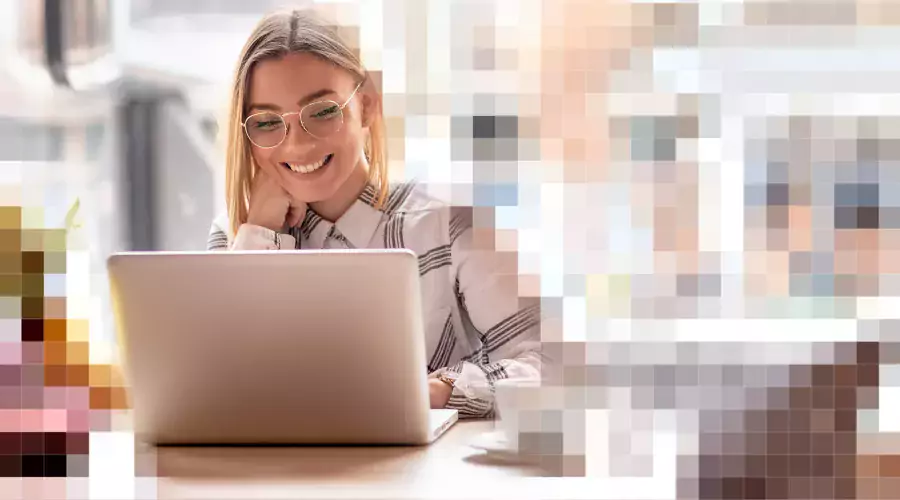
More about generative AI and iFinder Enterprise Search
The author
Franz Kögl
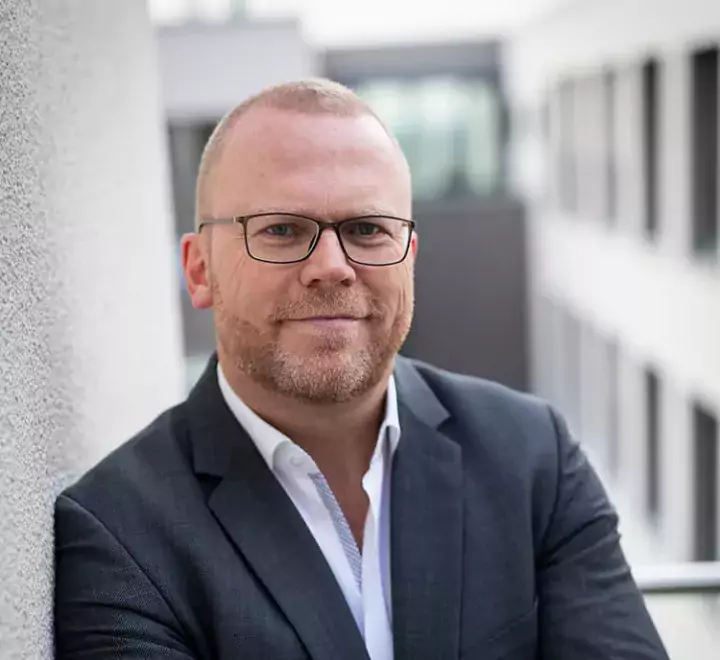