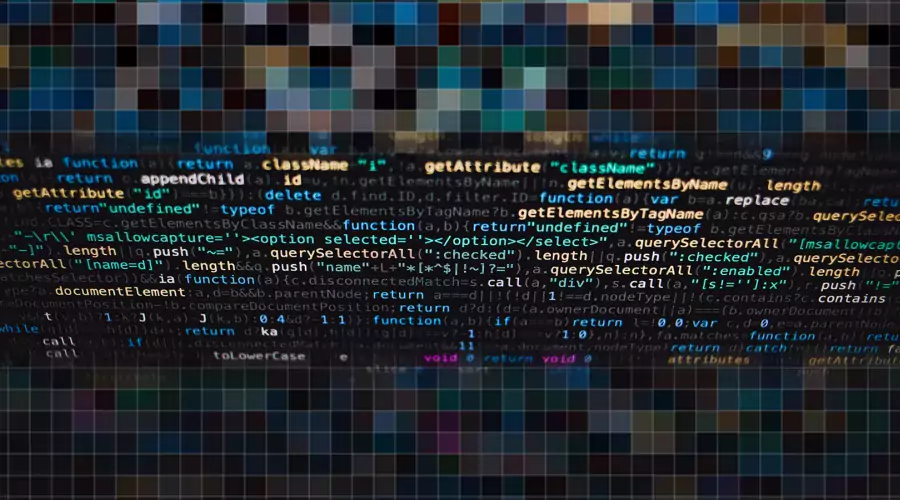
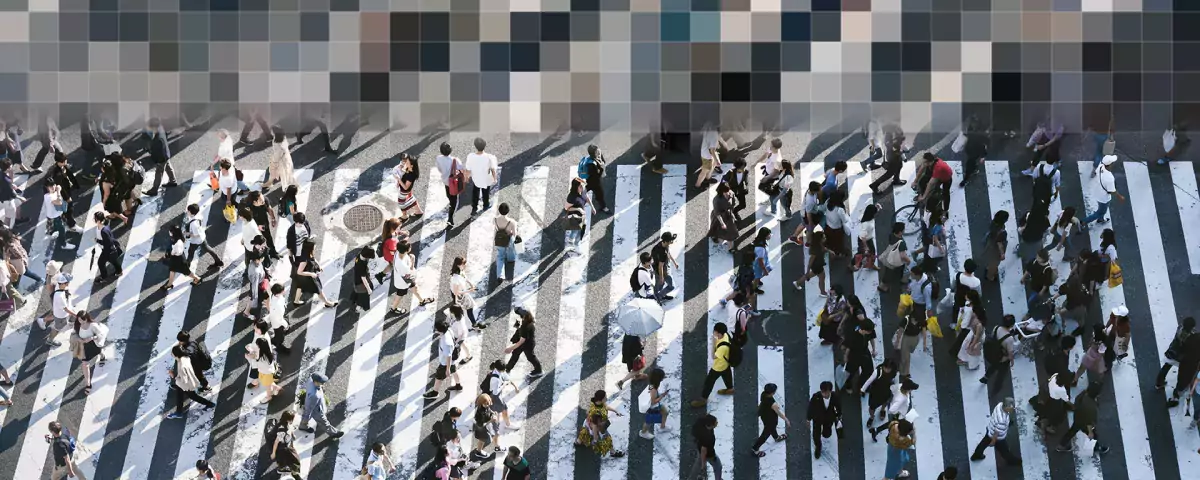
25.02.2021 | News AI for everyone? Transfer learning is a first step
Experts have high hopes for transfer learning, which involves using pre-trained models from other contexts to improve artificial intelligence. This is intended to solve one of the biggest problems AI faces when it enters real life and wants to prove its market maturity: the training data. They are always the bottleneck.
In principle, transfer learning is based on deep learning. This machine learning method uses neural networks to analyze data sets. Artificial neural networks are inspired by the biological neural network used by the human brain. Deep learning is used to recognize patterns, make predictions, or extract statistical properties from data. Real-world use cases include identifying and classifying objects in images or calculating suggestions for a text input. However, while humans tend to be generalists, neural networks can only solve very specific problems - namely, those for which they have been trained. Accordingly, a network that identifies objects in images cannot provide suggestions for text input. On top of that, neural networks need a great deal of training data, which subject matter experts must have annotated beforehand and which must then be cleaned up by AI experts and put into a format that is compatible with what the network expects. This makes them quite an expensive problem solver for the majority of companies.
Transfer learning can remedy this situation and make an important contribution to the widespread use of AI in the corporate world. Put simply, transfer learning is based on the idea that one machine learning system passes on its findings and its learned data model to another. In this way, the data can be used to analyze a similar type of problem. Two real-world application scenarios illustrate the potential: In document management, a general language model is used for recognizing specialized topics and is trained or adjusted to a specific subject area. Thus, only a few documents are needed for classification, for example, recognition of the document type, since prior knowledge in the form of general language skills is already encoded. AI-based contract analysis, on the other hand, which is based on software pre-trained for the most common types and clauses, can recognize agreements and key data points in contracts or tenders and extract them for targeted review, risk analysis, commenting and editing. Specialist lawyers, for example, save a lot of time with this intelligent reading aid, as they no longer have to go through contracts one by one and manually mark relevant passages.
The fact is, "normal" AI has always worked particularly well so far when companies like the big platform providers have a lot, even a lot, of data and use customized models. Google's image recognition, for example, solves a narrowly defined problem: classify images. So it can distinguish Chihuahuas from other dogs. Similarly, Internet translation services such as DeepL work thanks to a narrowly defined problem - in that case, translation - Deep Learning and a large amount of data. However, most companies beyond these technology giants lack the mass data needed to do this, for one thing, and the activities are usually very diverse. It is therefore practically impossible to collect the appropriate training data for each individual task, adapt a model to one's own requirements, and provide it to employees as a ready-made system. This is precisely where transfer learning comes into play. If the AI can access an already finished model, much less data is sufficient for further processing.
Of course, transfer learning is not a panacea: data is still needed, and the more varied the activity, the less likely it is that processes will be fully automated. Nevertheless, there are numerous ways to noticeably relieve the user of routine tasks. With pre-trained products from IntraFind, for example, task-specific solutions can be implemented very quickly, which also deliver a rapid return on investment. Transfer learning is therefore another important step in spreading the use of AI in the corporate world.
Related Articles
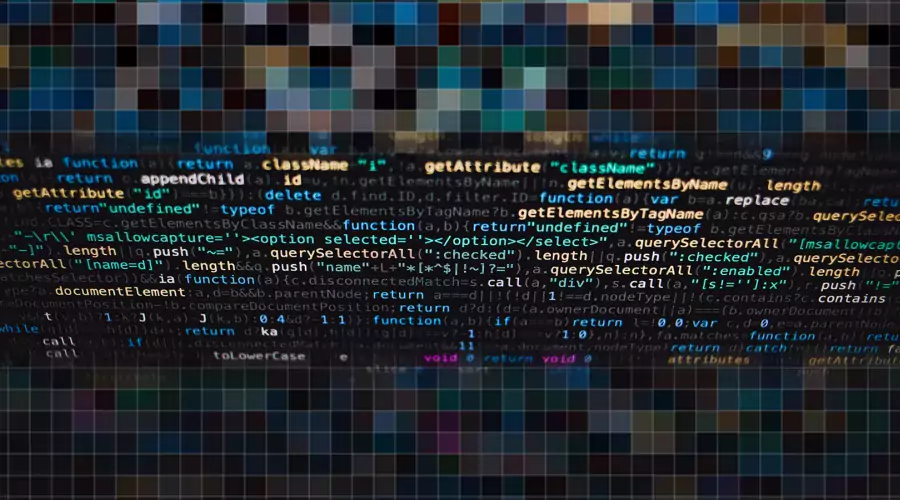
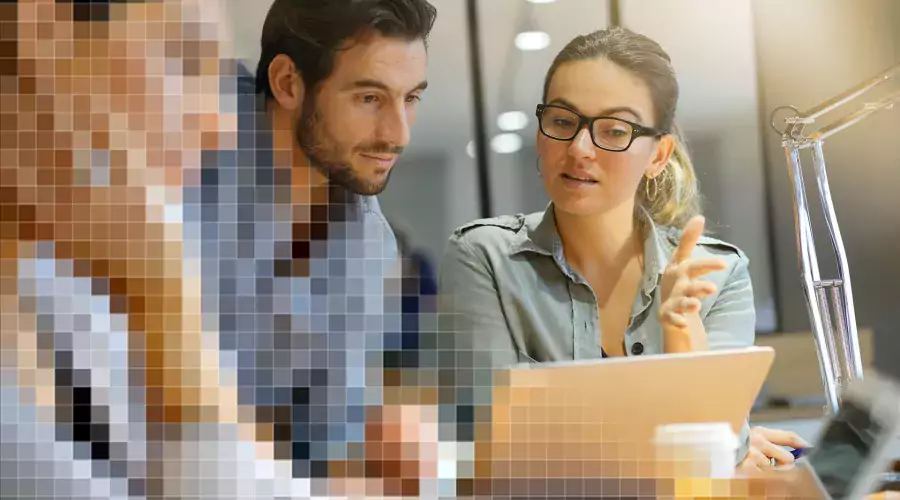
Artificial intelligence augments human intelligence
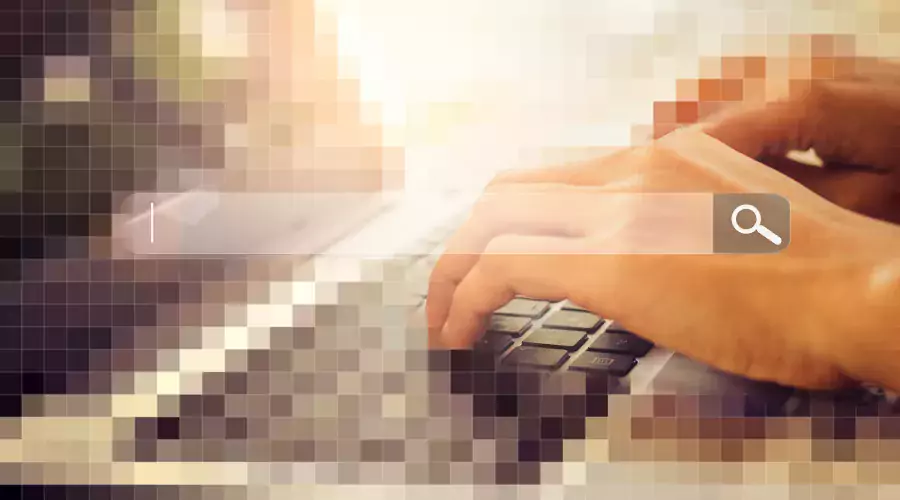