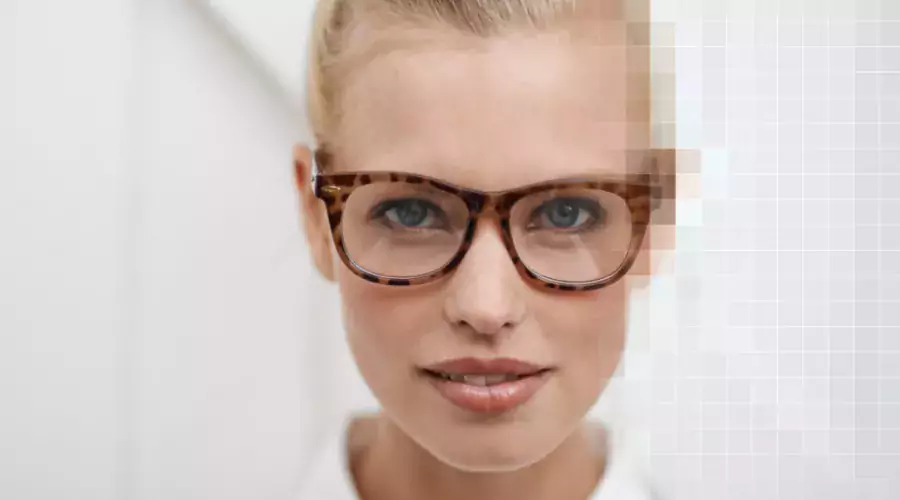
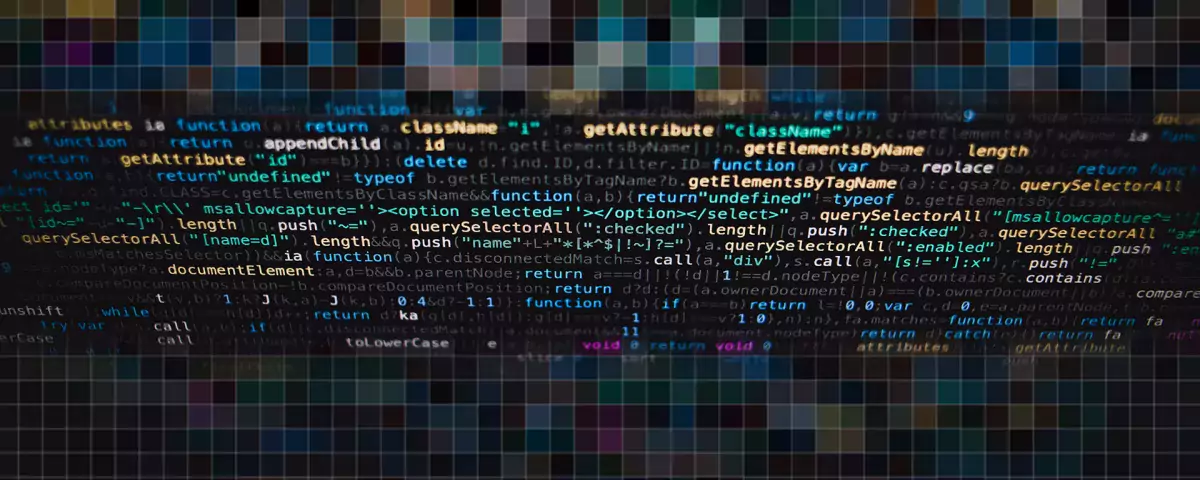
13.01.2021 | Blog Is Artificial Intelligence becoming a commodity?
In the field of machine learning, there are now countless open source frameworks - Spark, TensorFlow, PyTorch and many more. These developments in the field of open source make it increasingly easy to try out state-of-the-art machine learning methods, which is generally a good thing. However, this also raises the question of whether AI is now becoming a commodity and whether machine learning will soon be available "off the shelf".
However, there is still a long way to go. In the real world, data is often missing and the data you have is usually far from being "clean" or even fitting into the intended data model. Most of the effort in a machine learning project is to formally specify the problem, then obtain and clean the data for it, and then of course get a useful evaluation of the results.
In addition, the integration of the machine learning component, once it has been written or trained, is extremely complex. Thus, the formalization of the problem and the preparation for the work of the learning machine as well as the integration of the result into a workflow within the company are the most important and costly points of an ML project. Taking an ML model productive is then the very last and least costly step.
This means that technology is only half the battle in such projects. Successful machine learning projects need, above all, experienced experts who know how to use this technology to get the best out of the data they have available.
AI in the enterprise
From our private interaction with technology products, we have long been used to dealing with pre-trained systems/models. This includes, for example, the language recognizer in a cell phone, an automatic translator, or a photo app that recognizes that a person is being photographed and adjusts the camera settings accordingly.
These applications, created with machine learning, are built on large and most importantly available data sets and resources. These are pre-trained models that solve a narrowly defined task. The large amount of training data behind this is often also supplied to the app providers by our own use as customers.
Why, however, does AI, as we know it from the private world, not seem to arrive in the working world to the same extent? The answer is: Because you cannot have perfect pre-trained models for every workflow in different companies. From our point of view, artificial intelligence will not be able to quickly initiate the respective following process, such as an SAP workflow, at all companies in the world on the basis of an incoming order in any form - be it a simple email text, Word or PDF attachment. There is not enough training data for this because each company has its own processes and customized systems. It is therefore difficult to create a model for such use cases.
What can help companies here, however, are not ready-trained machines, but machines that can learn. To democratize AI more in companies, we also need machines that learn with the small amount of data that is available. Here, we can use transfer learning, a recent development from the field of deep learning, to learn quickly even with small amounts of data.
IntraFind Software AG, for example, masters classic machine learning and also relies on transfer learning or a combination of both, depending on the task to be solved. The software provider's goal is to help companies teach a machine what they need from it. One example of such a machine that can learn is the Contract Analyzer. Here, the software is pre-trained to recognize and extract specific clauses and data points in contracts. However, users can easily retrain the tool for other data points for their individual purposes.
AI at IntraFind
Related Articles
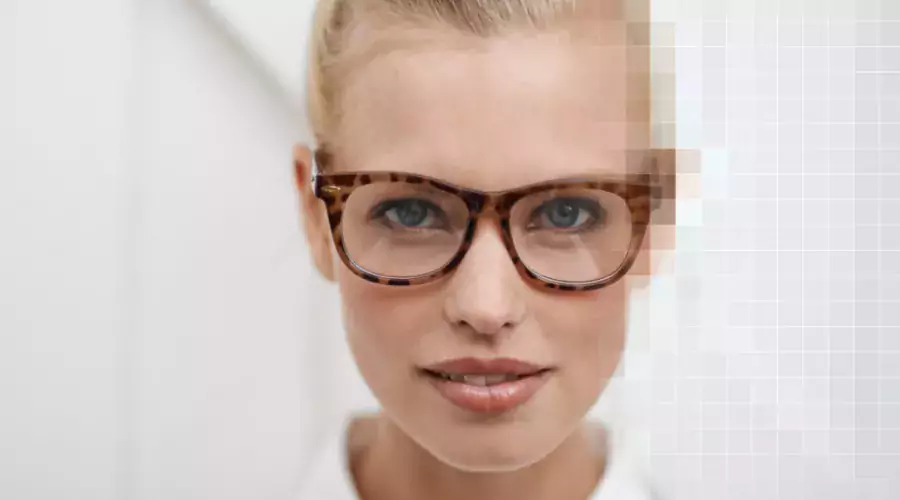
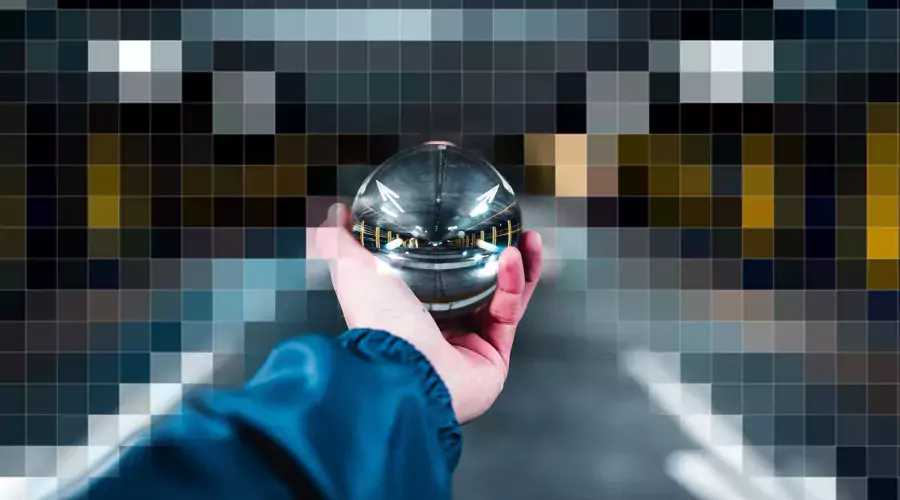
From the IntraFind blog: AI does not have magic powers
The author
Breno Faria
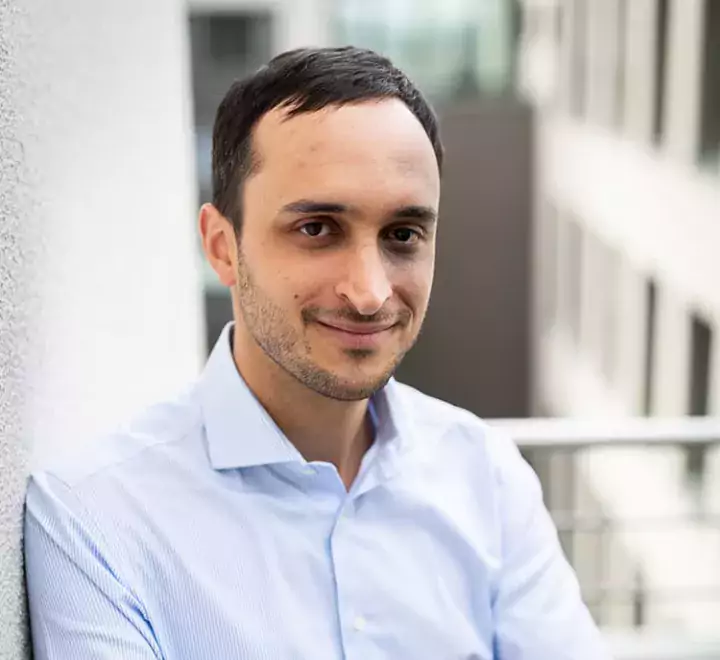