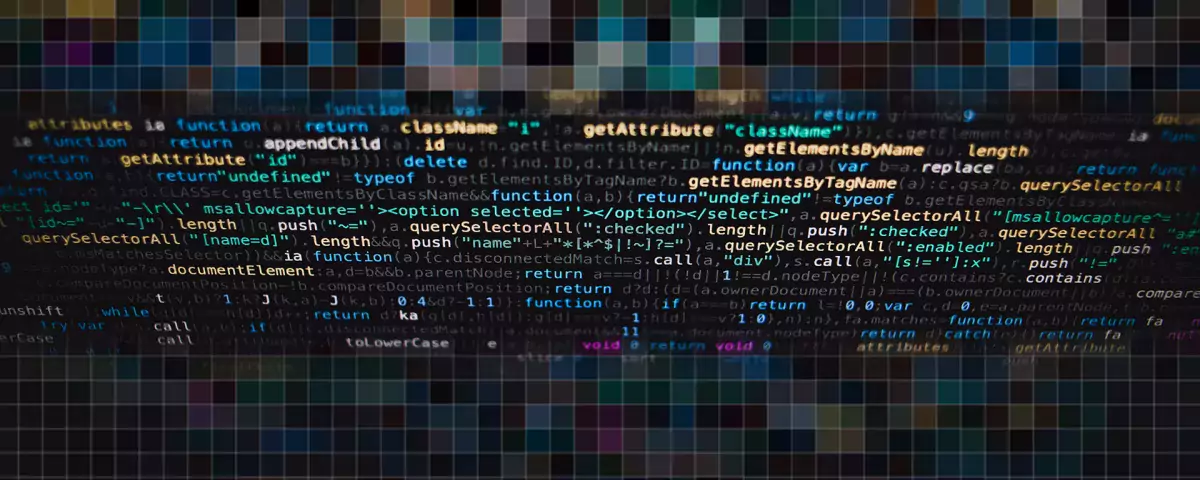
21.07.2020 | News Transfer Learning democratizes and simplifies AI usage
Transfer learning can accelerate the learning of a task by means of generic knowledge. This means that less data and computing resources are required to achieve good quality of the desired work results.
With the machine learning method transfer learning, neural networks that have already been pre-trained for a certain purpose can be used as a starting point for a different task. This approach makes it possible to use what has already been learned from a trained network for a new project. Thus, computationally intensive and time-consuming activities for training a new neural network are avoided. This method offers advantages, for example, when using "big data" models to solve "small data" challenges, especially in the area of processing text data using Natural Language Processing (NLP).
Transfer learning can make an important contribution to the democratization of AI, that is, the widespread use of AI in the corporate world. Up to now, AI has always worked particularly well when a company holds a lot of data and uses tailor-made models. Google image recognition, for example, can differentiate Chihuahuas from other dogs in images because Google has the necessary amount of data and can tailor models specifically for this type of image classification. Internet translation services like DeepL also work well thanks to neural networks, deep learning and a large amount of data.
"Despite these undeniable achievements, most office jobs are not simplified much by AI", explains Franz Kögl, CEO of IntraFind Software AG. "The reasons for this are that on the one hand the mass data is missing and on the other hand the office activities are very diverse. It is therefore practically impossible to collect large amounts of data for each individual activity, to tailor a model and to give it to the employees as a ready-to-use system. This is exactly where transfer learning comes into play.
Transfer learning achieved great success in the field of image processing and has therefore been increasingly used for language models since 2019. With the technology of transfer learning, pre-trained language models with relatively little training data are adapted for other tasks on the basis of comprehensive language understanding. Language models such as BERT (Bidirectional Encoder Representations from Transformers), ULMFiT (Universal Language Model Fine-Tuning), GPT and the enhancements GPT-2 and -3 (Generative Pretrained Transformer) have already proven that the information learned from one data set can be used for tasks in other data sets quite easily. Two specific application scenarios show the potential of transfer learning.
Successes in customer service and document management
In customer service, language models as well as voice and text analyses can be used to automatically forward customer calls or e-mails based on the customer's wishes. For example, it is possible to record support queries via virtual assistants, compare them with FAQ databases and then respond quickly.
And in document management, using the transfer learning method, a company does not have to start from scratch when identifying specialist topics. Instead, a general language model is used and trained or adjusted to a specific subject area. This means that fewer documents are required for classification, since previous knowledge in the form of general language skills is already coded.
"IntraFind has the goal to support companies to teach a machine what they need from it. With transfer learning methods and a generic learning machine, we have to make concessions regarding customized models and complete automation, but the workload of the users is reduced significantly", emphasizes Franz Kögl. "With our systems and solutions, for example, forms are not filled out automatically, but users receive all the information they need for this activity, for example by highlighting important passages of a document".