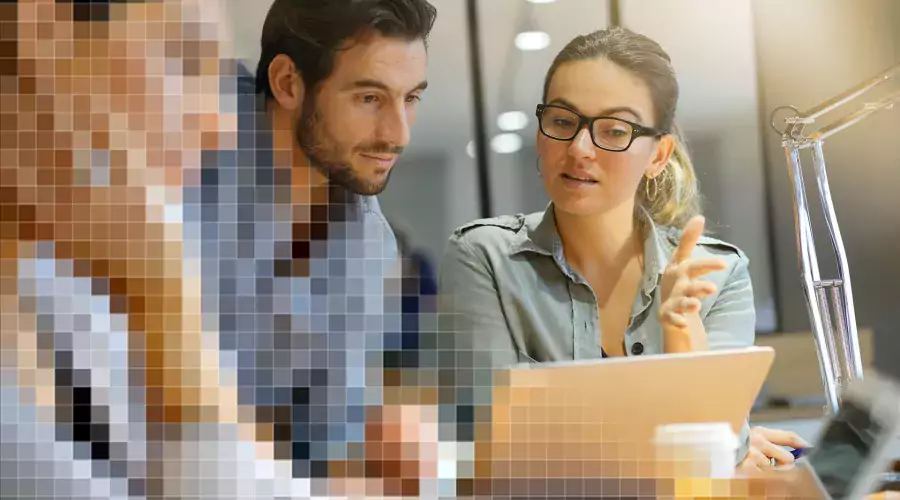
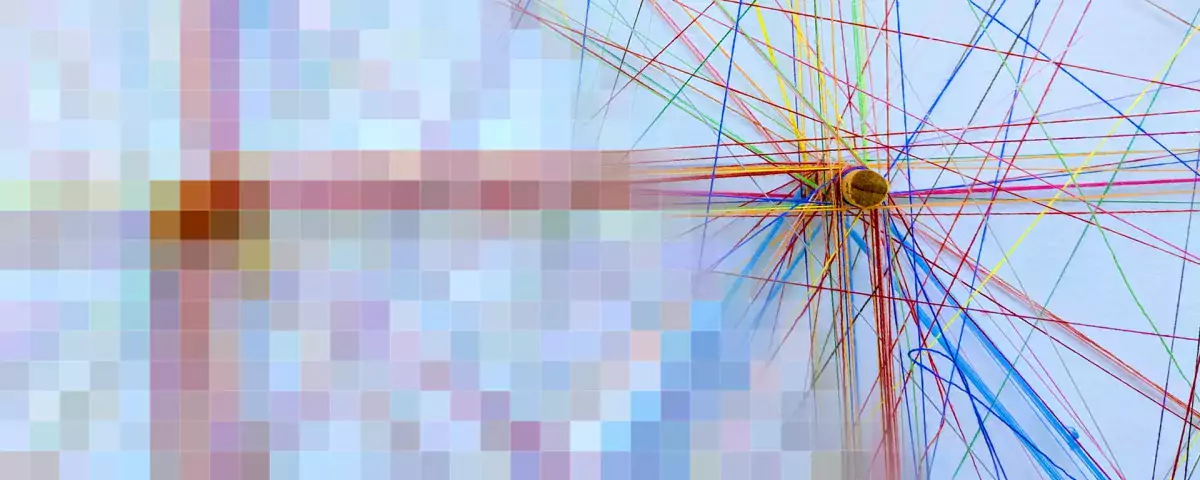
07.08.2020 | Blog Transfer learning - rapid success with little data
Transfer learning is a Deep Learning method. A pre-trained artificial neural network is used to solve new problems. Because transfer learning enables fast and resource-saving success with new tasks, it is an important tool for using artificial intelligence more easily and in a larger number of use cases.
AI works well for those companies that have a lot of data and can have models tailored to their needs: DeepL has a powerful translation service because the company has collected data and has invested heavily in its translation process. Google can distinguish Chihuahuas from other dogs in images because they have the data and can tailor models specifically for this type of image classification. Automobile manufacturers use large amounts of data to develop and perfect autonomous driving systems.
Does AI also work without mass data?
Despite these successes, most office jobs are hardly ever simplified by AI. Why is that? It is not only because of the lack of mass data, but also because office jobs are very diverse. It is therefore practically impossible to collect large amounts of data for each individual activity in a company, to tailor a model, to give it to the knowledge workers as a ready-made system like a speech recognizer, and to tell them: "From now on, you don't have to fill out this SAP form, AI will do it for you".
What is Deep Learning?
Practical application examples for Deep Learning are fully automated call centers, self-propelled cars or face recognition in photo programs. NLP - the understanding of natural language search queries and the output of corresponding results - is also an example of a Deep Learning application.
IntraFind, as a specialist for enterprise search and content analytics, therefore pursues the goal to provide knowledge workers with this tool and to teach the machine what the users need from it. Our software learns in practical use in the field to help people. The optimizations are not built in a priori. Rather, the system must function as a generic learning machine, so to speak.
Transfer learning therefore comes into play when you want to accelerate the learning of a task through generic prior knowledge. Let's take the example of contract analysis: Let's assume that the activity requires the recognition of home office clauses in employment contracts. Instead of starting from scratch when identifying specialist topics (What are letters? How do words differ syntactically, morphologically? etc.) it makes sense to train a system from a certain level of knowledge. That is, you take a pre-trained model with a comprehensive understanding of language that already recognizes sentences and basic forms of words etc. Then you train or adjust it with relatively little training data to a specific subject area, where it is then able to recognize connections. The recognition of home office clauses is thus based on an already functioning language model. This prior knowledge is already coded and therefore less documents are needed to train the ability to automatically recognize corresponding clauses and regulation text passages.
Transfer learning as a tool for the democratization of AI
Transfer learning, which originally comes from image processing, has also proven to be a sustainable method for language models. With relatively little data and fewer computing resources, this technique can help to produce high-quality models that would otherwise require huge data sets and enormous computing power. This sounds promising and simple, but in reality it is a highly complex process that requires the input of experts. Transfer learning will continue to develop and is already an important step towards enabling the increased use of AI in the corporate world - in other words, democratizing AI.
Related Articles
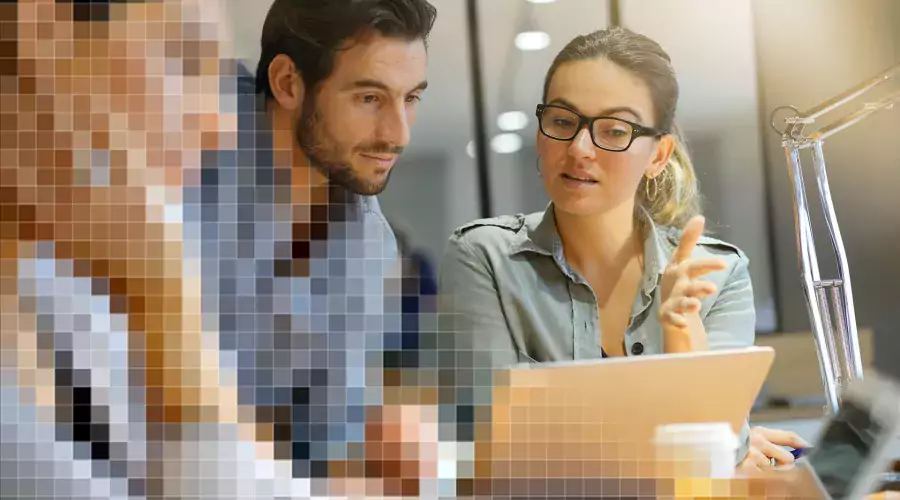
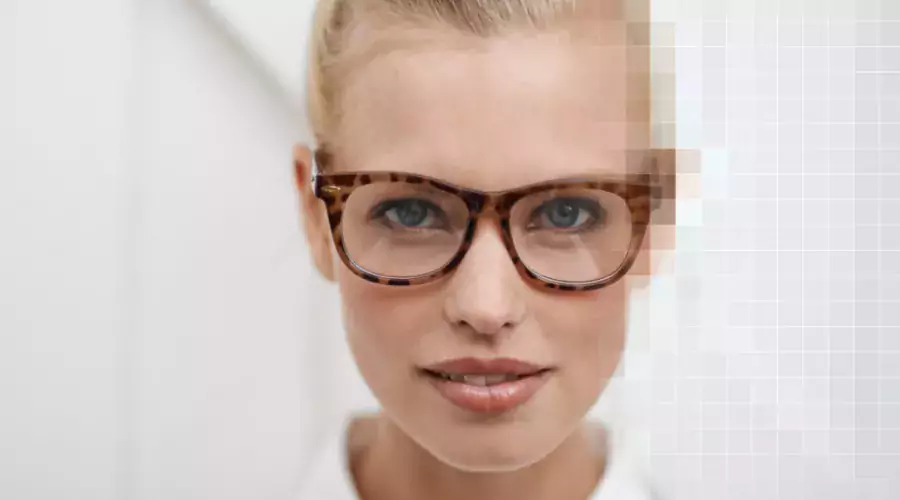
Product Deep Dive: Analyze Documents Intelligently with AI
The author
Breno Faria
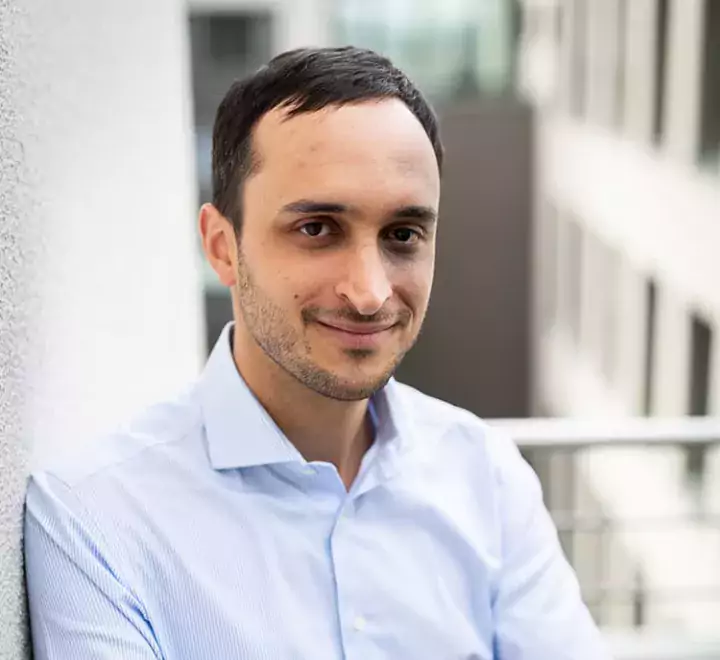